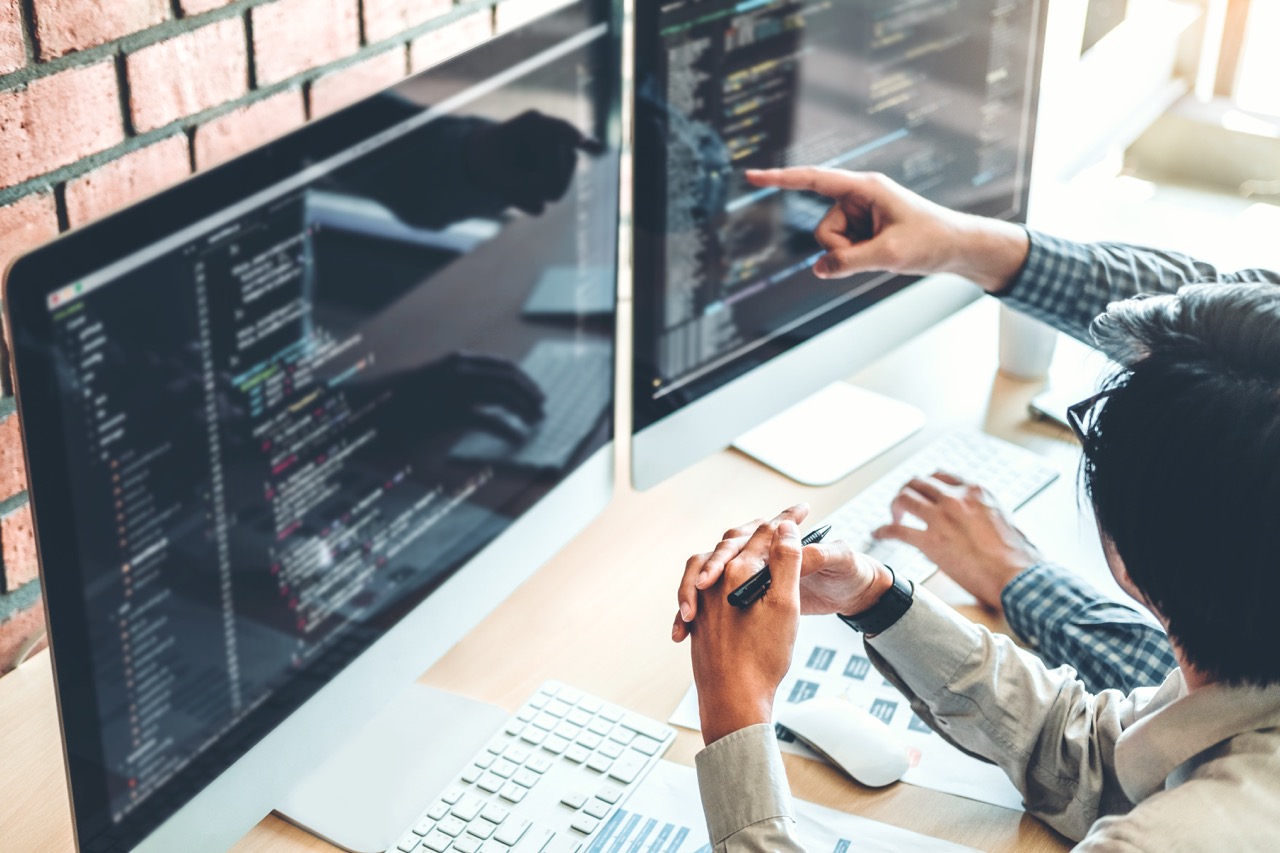
In Part I of this series, I have outlined some general considerations that could be summarized as discussing the spirit of the review process. In this article, I shall try to dissect and show you what a review might look like in practice – we shall examine the anatomy of a review, if you will.
Practical Guidelines for good reviews
Have a formal checklist
While I have stressed the informal part of a review more in Part I, it can help tremendously if the team can come up with a list of todos regarding every review. This checklist can then be referenced during every review. This should be an organic, ever-evolving list: if the team finds that certain items on the list become obsolete or want to add new ones, the checklist can and should be changed. Below are examples of what might be on such a list:
- read the user story together at the beginning of a review
- the reviewer should check out the feature branch himself and verify personally that the feature works
- the reviewee should do a mini-demo of their feature
- check for the existence of unit tests
- changes conform to our code standards (linting, naming conventions, etc)
What’s the ideal review size?
Ideally, reviews should be as small as possible, while still dealing with a standalone, self-contained increment. Even if the changes will eventually be deployed along with some other changes (e.g. because a user story needs multiple changes to happen to function properly), these changes can and should be reviewed separately. If it makes sense, you can have mid-feature reviews for the same user story – and with each additional review, you should focus on the new changes, naturally.
How long should a review last?
In my humble opinion, it is more useful to have a minimum length rather than a maximum length for reviews – especially when a team first embarks on having a more formalized review process. My recommendation is thus that the team should spend at least 15 minutes discussing the changes – even if they think the changes are trivial, more often than not when examined deeper it can prove to have more depth than one had first expected.
Over the long term, the team should try to anticipate and estimate how long a review process is expected to take for each user story. This obviously depends on a lot of variables (like feature size and number of people that need to be involved) – and also on whether or not the changes are approved or rejected -, but by thinking about the review step consciously during the estimation steps, this uncertainty can become more manageable. It also helps to have an explicit subtask for the review step that automatically gets created for each user story, so as to remind everyone during estimation that the review process will take time and to enable people to log their hours during development.
How many reviewers should there be?
Obviously, there should be at least 2 participants – the reviewee, and the reviewer. More are quite welcome, though: as long as they feel like they can actively participate and add to the conversation. If the changes in question are relevant for everyone, it might make sense to have an “all hands on deck” review as well (but those instances are generally rare).
Two things to note here:
- If you find that there is an overabundance of very active developers that want to be there for all the reviews, and you note that this is detrimental towards other development efforts, then first of all, you should tap yourself on the shoulders for having such engaged devs, and second, feel free to specify a max amount for people in each review.
- As a reviewer, it is completely acceptable to not stay for the entire length of the review. If you feel like you have exhausted your usefulness – have provided the best comments that you could, gave feedback you could, and/or are unable to participate according to the spirit of the process – feel free to say bye and log off. Just don’t forget to approve or disapprove based on what you saw.
Where should the review take place?
Ideally, reviews should be done synchronously – by that I mean “in person”, or “online in a meeting”. Reason for this is that reviews should facilitate two-way communication, and some of the best results can only happen in this sort of environment. Every member of the team should know when reviews take place, and participation should be easy and open for anyone. This might be the most controversial of my takes, because developers tend to be more introverted, and some might find this to be slightly outside their comfort zone. Barring some insurmountable obstacles like huge time zone differences however, this is indispensable for the process to work well.
Common objections
Finally, let’s discuss some common objections one might have when first introducing regular reviews. I found the following two to be the most salient:
1. “We don’t have enough time!”
The problem with this argumentation is that it basically proposes that you can actually save time by skipping this step – but the exact opposite is true.
First of all, errors caught during the review step cost way less time and money to fix than anywhere later in the development cycle. Fixing a bug in production will at the very least involve creating a ticket, writing a ticket description, pulling a dev off of something else they could be working on, having them investigate the issue, then hopefully once they have identified it, fixing it, then waiting for the next deploy cycle to happen till it can be fixed. And speaking of which, would you rather have an end user or a client find the issues, or one of your devs?
Second, in any ongoing development effort, there will come a point where someone will have to work on part of the code that they have not written themselves. When (not if!) this happens, they will have to spend a lot of time doing codewalks with the original author(s) anyway – and God help you if they aren’t available anymore, in which case you can expect to have tickets called “Code Archeology – 3 days” pop up on your board, just so people can understand what certain parts of your codebase are doing.
2. “Noone else can really understand, and thus, review my code”
It might happen that there is only one person on the team who is an expert in one particular field – especially if they are a senior in their field. If this is the case, it is tempting to let them roam free, and accept that there is just no way to feasibly review their changes – after all, they are an expert.
This approach, however, would be a fatal mistake. Even if the colleague in question writes flawless code, this should raise all sorts of alarm bells. A single point of failure such as this can eventually have disastrous consequences if said colleague decides to leave the company (or just goes on extended vacation or falls sick).
Instead of this, have someone else from the team, preferably someone who is open to learn something new, review their code nonetheless. Even if the reviewer will at first add little more value than being a rubber duck in the review meeting (which can be surprisingly useful in and of itself), they will end up asking a lot of questions and eventually, you will find that you now have 2 people on the team who can handle that particular field. If this is your situation, consider giving the second dev the easier tasks as they come along, and have them actively develop one part to speed up the onboarding process.
The importance of getting everyone onboard
Ultimately, the biggest hurdle you might face when trying to kickstart a healthy review culture is from within the team itself. If any one on the team does not see the value of a review, they will fail to conscientiously apply themselves at it, and will ultimately undermine the process at every turn. That’s why it is important to talk this over with everyone, explain how this will benefit them personally and the project as a whole, and assuage any unrealistic expectations or fears they might have (e.g. unrealistic fears of potential repercussions for failing the review process). Ideally, have everyone actively participate in creating the review process, so they themselves can own it and apply it for their own benefit.
In conclusion, having a review process for code changes is crucial for the success of any development team. In this article, I have outlined some guidelines for a healthy review process, and tried to address a few common objections I have encountered. (If you have some other objections that you have heard, feel free to drop me an email – I’m genuinely interested!)
I hope by this point you can plainly see that the benefits of having a thorough review process far outweigh any perceived time constraints or inconveniences, and by utilizing it, development teams can improve code quality, communication, and ultimately, project success.
Legutóbbi hozzászólások